Reducing the complexity of design space exploration with sensitivity analysis
Written by Danilo Di Stefano and Alessandro Viola
10 March 2021 · 6 min read
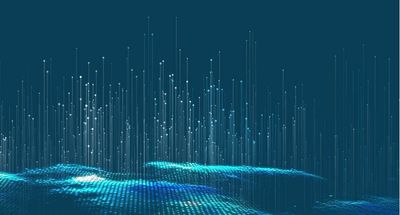
Engineering problems can involve a large number of design variables. But not all of the inputs have an equal effect on the output. Considering them all can dramatically reduce the efficiency of Design of Experiments (DOE) or optimization studies. In this scenario, you can also experience difficulties when building Response Surface Models (RSM). Most probably your dataset includes needless parameters which make your RSM training unnecessarily more complex.
On the other hand, your team of CAE optimization engineers and analysts may disagree about which parameters affect your design problem the most if these decisions are made manually. The human mind can manually grasp up to several key effects of what is driving the system. This leads to uncertainties about the interaction effects between design variables and some lower or lesser effects in your system.
Defining the influence of parameters on the outputs of your simulation model before undertaking design space exploration studies can reduce the complexity of your assignment. Excluding input variables with negligible effects allows to reduce the size of the design space, and lets the intelligent algorithms find optimum solutions faster, without losing model accuracy or reliability.
Understanding how design parameters affect responses before performing optimization enables you to reduce the complexity and find optimal solutions faster.
However, sensitivity analysis itself can be a very time consuming task, potentially requiring many design evaluations. Variable screening methodologies are able to extract sensitivity information with a relatively small number of sample points, this way reducing the computational cost of sensitivity analysis.
Sensitivity analysis: rank parameters in your engineering design problem
With sensitivity analysis, you can identify key independent variables impacting performance metrics, and the quantitative nature of their impact respectively. If an independent variable is not heavily impacting any of the performance measures, it can be fixed to a nominal constant value, thereby increasing the efficiency of your exploration and optimization task.
How can you practically investigate the robustness of the model predictions? Our process automation and design optimization software, modeFRONTIER, comes with advanced simulation data analysis tools including sensitivity analysis. It helps you to explore the design space in a meaningful way and to assess the statistical significance of the obtained results from dedicated experiments.
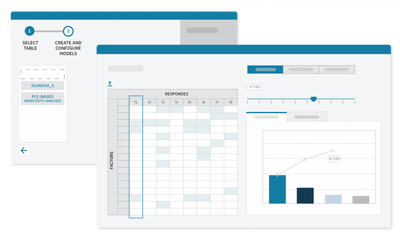
Perform sensitivity analysis with modeFRONTIER
The Sensitivity analysis tool in modeFRONTIER includes SS-ANOVA and PCE-Based sensitivity analysis methodologies:
- Smoothing Spline ANOVA (SS-ANOVA) is a statistical modeling approach based on a function decomposition similar to the classical analysis of variance (ANOVA) decomposition and the associated notions of main effects and interactions. Each term –main effects and interactions– exhibits the measure of its contribution to the global variance (so its relative significance). SS-ANOVA is a suitable screening technique for detecting important variables in a given dataset.
- PCE-based sensitivity analysis is a variance based approach to sensitivity analysis. It relies on Sobol indices, intended to determine how much of the variability in model output is dependent upon each of the input parameters, either upon a single parameter or upon an interaction between different parameters. Polynomial chaos expansion is used to reduce the number of samples needed to calculate Sobol indices.
How it works: Filter out unimportant factors with the Effect table chart
Effective visualization of contributions can play a significant role in sensitivity analysis, especially when many factors are involved. The new Effect Table dashboard available in modeFRONTIER shows you in a single place all the information you need to get informed decisions.
The effect table chart shows the relative significance of different terms - that is the percentage of contribution of each term to the global variance - and enables you to choose and adjust a screening filter to exclude the most unimportant terms. Bar charts can quickly display the relative contributions of factors. Sliders can help to identify the most promising overall conditions, by quickly displaying side modifications between factors.
When you apply the filter in the chart, they are saved in the Sensitivity model. Then, your sensitivity model is ready to be used automatically in modeFRONTIER:
- In the RSM training to automatically exclude unimportant inputs from the training of RSM models for each output.
- In the input domain definition to transform unimportant input variables into constants
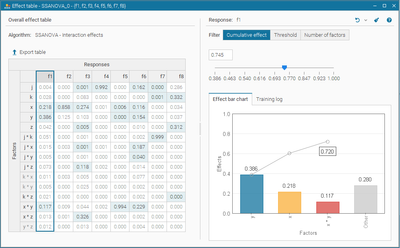
Sensitivity analysis comes in a wide range of industry-specific engineering problems
The benefits of combining design space exploration with sensitivity analysis are clear. The automotive, aerospace and other industries employ this approach to reduce engineering problem complexity, and consequently their development timescales, across a broad range of use cases.
Along with the spread of simulation in other less "traditional" industries like architecture and construction, sensitivity analysis can also be applied in this field. For example, researchers from the Delft University of Technology performed sensitivity analysis in modeFRONTIER to define which construction parameters have the highest impact on annual energy demand and thermal comfort for a nearly Zero-Energy high-rise office building. From the sensitivity analysis dashboard available in modeFRONTIER, they could visualize the impact of these variables on both energy use and thermal comfort.
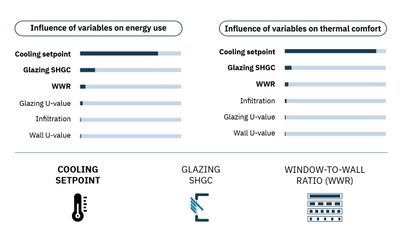
The sensitivity analysis shows that the cooling set-point has the highest influence, followed by SHGC (Solar Heat Gain Coefficient) and Window to Wall Ratio (WWR). Glazing U value, infiltration rate, and wall U value appear to have minimized influence on these objectives. These assumptions make the optimization strategy more efficient when investigating the influence of building parameters that can have contradictory impacts on cooling, lighting, heating energy loads, and facade orientations.
In summary, when approaching an engineering problem it is advisable to use sensitivity analysis to reduce its complexity. It allows you to reshape the design space into a more rational one and then improve the quality of the results achieved with DOE, RSM training, and optimization studies.
Learn how sensitivity analysis helped Lucid Motors, Bottero and Luna Rossa Challenge when approaching multi-objective design optimization.
modeFRONTIER is the leading software solution for simulation process automation and design optimization
Design better products faster
modeFRONTIER is the leading software solution for simulation process automation and design optimization
Design better products faster
modeFRONTIER is the leading software solution for simulation process automation and design optimization