Speed up the development of EV battery cells with parametric design optimization
Written by Apurva Gokhale and Michael Bambula
24 September 2024 · 5 min read
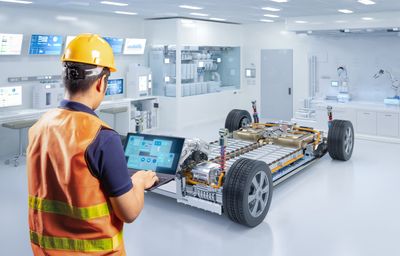
The global trend towards electrification, particularly with the rise of electric vehicles (EVs) in the automotive industry, has created a growing demand for lithium-ion batteries. This is a relatively new domain and presents significant challenges for original equipment manufacturers (OEMs) and pack suppliers who often lack expertise in the chemical manufacturing process involved in making these batteries.
At the heart of lithium-ion batteries is the cell, the fundamental building block. Designing an optimal cell requires a thorough understanding of its requirements, which includes delivering high energy density, maintaining stable voltage output, ensuring a long cycle life, and demonstrating excellent thermal stability. It’s important to design a battery cell that can maintain required performance even when subjected to manufacturing tolerances.
To address this knowledge gap and improve the cell manufacturing process, we propose a novel simulation-driven approach which combines the AVL multi-physics system simulation solver with the modeFRONTIER process automation and design optimization software.
Overview of lithium-ion battery manufacturing process
Our simulation-driven design approach helps to identify optimal cell designs and predicts their performance in view of manufacturing tolerances. But, before we get into the specifics of our approach, let's take a look at the stages of the manufacturing process for lithium-ion batteries relevant to our simulation analysis:
- Dosing and mixing of the electrode slurry.
- Coating and drying of the electrode foil.
- Calendering of the electrode in a rolling mill.
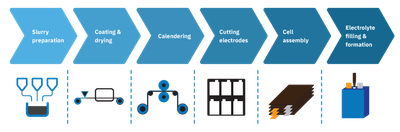
The key steps of lithium-ion battery manufacturing process
The dosing and mixing of the electrode slurry is a crucial step in the manufacturing of cells. The electrode slurry for anode and cathode, consisting of active material, binder, additives and solvents, must be carefully blended to ensure the uniform distribution of these components. Proper dosing of the raw materials is essential to achieve the desired electrochemical performance of the battery.
The electrode foil is then coated with slurry using a slot die to ensure distribution. Monitoring the coating of the foil is critical to achieving the desired porosity and layer thickness. The coated sections are then sent through a drying channel to remove any excess moisture and solidify the coating.
Lastly, to ensure the uniform thickness of the electrode, which is essential to performance, the lithium-ion battery undergoes calendering. The foil is compressed with rollers and shaped into the final form. The roller pressure influences porosity and the energy density of the cell. An excessive roller pressure will cause stress cracks in the electrode.
Manage battery development with AVL advanced simulation technologies
Electrochemical experimentation for lithium-ion battery development is a time consuming process. It requires multiple hands-on steps to create a prototype cell and thorough testing which takes even more time. AVL is capable of virtualizing much of this process. It defines chemistries, assesses the resulting cell performance and analyzes the electrical, thermal, and safety characteristics of the battery system in a virtual environment — all at a fraction of the time and cost of the real experimental process. AVL offers comprehensive battery model variations in the AVL AST Toolset. Models can be simple, heat source models or P2D electrochemical models used in 1D system (CRUISE™ M) or 3D CFD (FIRE™ M) simulations. AVL’s physics simulation software also integrates with the Batemo Cell Library (from Batemo GmbH), allowing users to access highly accurate electrochemical models of named cells from manufacturers like LG Chem, Samsung, Sanyo, SKI and Toshiba, among others.
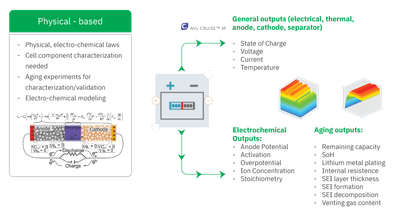
AVL CRUISE M Electrochemical Battery Model
The AVL CRUISE M electrochemical battery model is based on the Doyle Fuller Newman (or DFN aka P2D) model. In this model the cell is discretized along the x-direction (from anode to cathode), with the active material also being discretized radially. This is what gives it the name “pseudo-two-dimensional”. The advantage of using an electrochemical model lies in the fact that a battery’s performance is fundamentally a chemical phenomenon. By incorporating these chemical effects, users can gain insights into underlying processes such as anode potential and lithium plating which occur dynamically. Users can also access the sensitivity of cell performance of geometrical qualities, like anode and cathode thickness, as well as the particle radius of the active material. These insights are valuable for battery design and for BMS algorithm development. In this study we are in the early stages of the design process and focusing on how cell chemistry design is sensitive to manufacturing tolerances. The model we’re using is from our CRUISE M example library. It was developed internally at AVL and based on LG Chem INR18650-MJ1. It was developed using test data and literature sources.
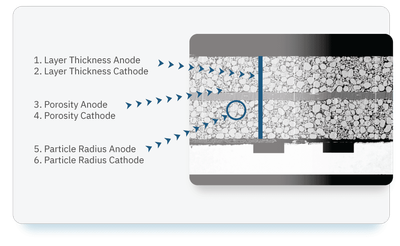
Design variable overview and cross-section view of electrode
In the study, we vary six parameters with characteristics that are sensitive to the early- stage manufacturing of the battery cell. The slurry preparation, coating and drying, and calendering processes impact the layer thickness, porosity, and particle radius of the anode and cathode. These parameters were selected to understand how deviations in the manufacturing process could ultimately affect cell performance.
To understand the impact of these variables on cell performance, we need to apply statistical methods like those found in modeFRONTIER. It’s also crucial that CRUISE™ M can be executed repeatedly during the variation study, a process seamlessly integrated with modeFRONTIER. Given the computational complexities involved, the ability to generate accurate meta-models (Response Surface Models) is invaluable, which modeFRONTIER also provides. Together, these capabilities in a cohesive workflow between CRUISE™ M and modeFRONTIER create a powerful tool for battery chemistry assessment.
Optimize a battery cell simulation model with modeFRONTIER
After creating a model of the lithium-ion battery cell, we used modeFRONTIER to automate the simulation of an AVL Cruise M model. This automated simulation process enabled us to run design exploration and optimization studies, where various algorithms modified design parameters and analyzed their impact on key objectives. Here’s a closer look at this optimization-driven design process.
1. Integrate AVL Cruise M battery model into an automated simulation workflow
We used an automation workflow in modeFRONTIER to conduct various studies on simulation models. We considered six design variables related to the AVL battery model, including thickness, porosity, and particle radius for both cathode and anode. We also defined the lower and upper bounds for these variables. modeFRONTIER automatically adjusted the design variables based on the selected algorithm and fed them into the AVL Cruise simulation model to solve one particular charging profile. Response variables (outputs) included anode potential, power loss, and maximum charge.
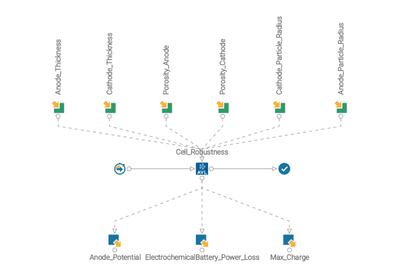
Automated Cruise M simulation model within modeFRONTIER workflow
2. Perform Design of Experiments (DOE) and sensitivity analysis
For this study, we began by analyzing the relationships between input variables and output metrics through a Design of Experiments (DOE). By running 120 designs, we were able to determine that the charge is highly correlated with most of the input variables, whereas power loss is primarily influenced by the thickness of the cathode.
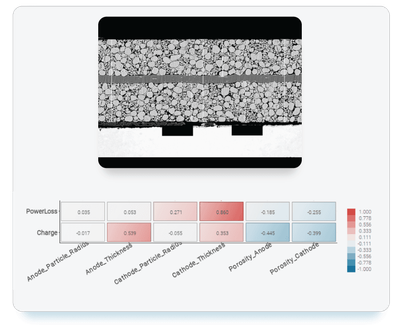
Sensitivity and correlations assessment
3. Apply design optimization to maximize charge and minimize power loss
Once we understood how input variables affect responses, our goal was to identify the optimal lithium-ion battery cell settings to maximize charge while minimizing power loss. These objectives were defined in the modeFRONTIER workflow. Given that power loss and charge are positively correlated (with higher charge leading to increased power loss), we set a constraint where the anode potential must always be greater than zero.
modeFRONTIER offers a variety of optimization algorithms, including the pilOPT, our proprietary multi-strategy algorithm. The software supports multiple objectives and constraints, making it well-suited for our design problem. With our goals defined and the algorithm selected, we then used modeFRONTIER to automate the optimization process and performed 500 simulations in just four hours. From the full set of Pareto front solutions, we selected the three designs that best balanced our objectives and constraints:
- Design 1: high charge, high power loss.
- Design 2: best compromise between charge and power loss.
- Design 3: low power loss, low charge.
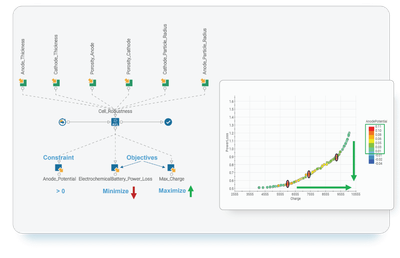
maximize charge and minimize power loss with modeFRONTIER optimization algorithms
4. Understanding variations in performance due to manufacturing tolerances
While the optimization study provided us with three design solutions, further analysis was required to ensure that cell performance remained within the expected range despite uncertainties in design variables caused by manufacturing tolerances. Given AVL’s expertise in both modeling and manufacturing process of cells, we were aware of the distribution and tolerances for anode and cathode thickness, porosity and particle size. Consequently, we treated all six design variables as stochastic and modeled them with normal distributions to simulate real-world variations. We wanted answers to these questions:
- What distributions for charge and power loss result from the variations of these design variables?
- Can we estimate the standard deviations of these distributions and ensure that the performance remains within acceptable limits?
- Given an acceptable range of performance for these responses, how can we determine an acceptable range for our input variables?
With modeFRONTIER, we conducted robust analysis to study design behavior in the presence of variations of input variables. We started from the three designs selected from the Pareto solutions obtained from the previous optimization phase. Leveraging AVL’s expertise, we determined the standard deviation for thickness, porosity and particle size for both the cathode and anode. We then performed adaptive sparse polynomial chaos expansion (PCE) sampling. We obtained 250 samples per nominal design which allowed us to perform background mathematical operations and then derive a probability density function (PDF) of charge and power loss. These samples, seen in the figure below, transformed three designs from deterministic optimization into three clusters of likely solutions. Thanks to robust analysis, we were able to select a design that offers the best tradeoff between performance objectives and minimizes variation in performance.
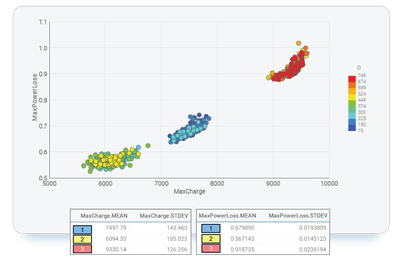
Manufacturing tolerances assessment with modeFRONTIER
In summary, we’ve developed a comprehensive approach to the parametric design of lithium-ion battery cells. With AVL Cruise, we modeled and simulated the battery, while modeFRONTIER automated AVL simulations, allowing us to conduct multiple design exploration and optimization studies. Our objective was to understand the sensitivity and correlations among design variables,optimize performance criteria while respecting constraints. Additionally, we performed robust analysis around the selected designs to ensure that charge and power loss performance remained within acceptable limits despite uncertainties in design variables. Here’s a breakdown of our next steps to expand the scope of the project:
- Incorporate additional charging profiles and different temperatures. These factors are critical in mobility and battery performance.
- Study the impact of aging on battery performance.
- Perform reverse multi-objective robust design optimization (MORDO) with modeFRONTIER to determine the tolerances for design variables required to maintain performance within the desired range.
modeFRONTIER is the leading software solution for simulation process automation and design optimization
Design better products faster
modeFRONTIER is the leading software solution for simulation process automation and design optimization
Design better products faster
modeFRONTIER is the leading software solution for simulation process automation and design optimization