Quality engineering based on the Taguchi robust optimization framework
Written by Mariapia Marchi
9 March 2020 · 7 min read
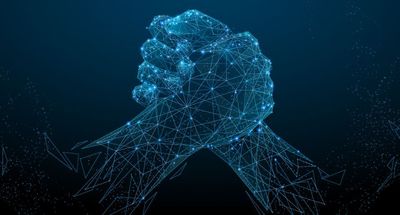
Defects, failures and, more in general, the poor quality of a product result in losses in users’ experience and consequent economical losses in the market. Quality engineering, a seminal robust optimization framework elaborated by the Japanese statistician and engineer Dr. Genichi Taguchi aims to tackle these shortcomings by predicting and preventing them.
The Taguchi method is defined as a series of approaches to predict and prevent problems that might occur in the marketplace after a product is sold and used by customers under various environmental and application conditions for the duration of the designed product life.
Quality engineering aims to predict and prevent problems that might occur after a product is sold and used by customers under various environmental and application conditions for the duration of the product life.
Taguchi assumed that a quality loss occurs when a quality characteristic deviates from its best or target value and that the loss is proportional to the square of the deviation. The effect of poor quality is assigned a value through the use of a loss model function. This means that the relationship between the quality loss and quality characteristic can be drawn as a parabola, with zero loss when the quality characteristic is equal to the target value.
The quality loss function also has a monetary interpretation: deviating from a product target performance has a cost, which increases quadratically with the distance from the target.
Taguchi’s method strives for zero loss, by identifying the important parameters that define a product and reducing performance variability for such parameters.
Robustness according to Taguchi: minimizing noise factors
Quality engineering aims at improving productivity during research and development stages to provide high-quality products, quickly and at a low cost. The goal is to guarantee a standard quality level for the entire product lifetime and for different application regimes.
According to Taguchi, “robust” design has a minimized function variability under different uncontrollable conditions (or noise factors). The idea behind this robust design is to improve the quality of a product by minimizing the effects of such variability without eliminating its causes (since it could be too difficult to determine them or too expensive to control).
The uncontrollable conditions that induce alterations in product quality are called noise factors. There can be external noise factors (like environmental conditions, vibrations, etc.), internal noise factors (like component deterioration, wearing, etc.), and noise due to “between product” variations. Robust design is little sensitive to variations in noise factors.
To determine whether a product is robust or not, we can analyze the so-called signal-to-noise ratio, which grossly represents the ratio of the mean performance (signal) over the standard deviation (noise). Signal-to-noise ratio should be maximized: the larger the signal-to-noise ratio, the smaller the variability around desired target values and the smaller the loss to the market.
To determine whether a product is robust or not, we can analyze the signal-to-noise ratio, which grossly represents the ratio of the mean performance (signal) over the standard deviation (noise).
The three stages of quality engineering
The Taguchi method for quality engineering can be seen as a three-stage process.
System design is the first stage. It is a conceptual phase. It identifies the important parameters that characterize a product and the quantities of interest.
The second stage is the parameter design. Parameter design assesses the product or process robustness, by means of an experimental analysis of system variables and functional requirements under various noise conditions. Parameter design is the most important step of the Taguchi method. The aim is to find the appropriate design factor levels to make the system less sensitive to variations in uncontrollable noise factors, without actually eliminating the variation causes. Parameter design allows quality improvements without requiring better materials or components, which means at no cost increase.
Tolerance design is the last stage of the Taguchi method. This step aims at minimizing the manufacturing and lifetime costs of products or processes by setting appropriate tolerance values for factors that have the largest influence on system response variation.
However, this step should be performed only if the quality target value has not been achieved during the parameter design stage. In fact, an inconsiderate tightening of tolerance values increases product and process costs.
The three stages of the Taguchi method for quality engineering process are: system design, parameter design, and tolerance design.
The overall goal of the Taguchi method is to find a good balance between product quality, i.e. the functionalities desired by customers, engineering quality, i.e. the reduction of functional variability, and production costs.
Need to change practitioners’ mindset
The origin of the Taguchi method dates back to the end of the ’50s, but quality engineering became well-established in Japan around the mid-’70s and was adopted by American companies in the ’80s.
Only recently, in 2014, parameter design became a standard ISO method: ISO 16336:2014 “Applications of statistical and related methods to new technology and product development process – Robust parameter design (RPD)”. The spread of the Taguchi method required a large effort from the practitioners’ side to understand apparently complex concepts and to change their mindset from the so-called goal post philosophy to a design approach based on the minimization of quality losses.
The advantages of the Taguchi method are highlighted by a popular study that compares the quality characteristic of the color density distribution of Sony color televisions manufactured in the USA and in Japan at the end of the ’70s.
The US color frequency distribution was uniform within the tolerance range, while the distribution for Japanese products was a normal distribution around a target value.
This implies that most of the Japanese televisions had a color frequency close to the target value and there was a non-null probability that a few televisions might have a color frequency out of the tolerance bounds.
In spite of the second implication, US buyers appeared to prefer Sony televisions manufactured in Japan.
Customers’ satisfaction was maximized by the Taguchi method approach of meeting a quality target value and minimizing the variation around that target, rather than meeting the tolerances as done by the US manufacturing approach.
Taguchi method and parameter design as an ISO standard
The exact procedure of the Taguchi method depends on the nature of the design problem, but it is possible to outline a generic sequence that constitutes the standard ISO 16336:2014 procedure. The ISO addresses two main use cases: 1. robustness assessment through signal-to-noise ratio; 2. parameter design
The first use case aims at assessing the robustness of a single design for fixed control variable values.
The second use case extends the first. Several designs are assessed with the aim of optimization and decision making. Here, optimization is not an automated process, but it requires manual operations: after selecting the best design concept on the basis of SN ratio values (first use case), designers should perform a detailed design optimization by selecting optimal nominal values of control factors in such a way to adjust the response to a target value (by checking sensitivity values).
In practice, parameter design is a two-step optimization procedure that first maximizes product robustness (insensitivity to noise) and then adjusts the performance level to a target value.
The portfolio of robust design optimization tools of ESTECO products has been complemented with a new module for quality engineering which allows taking advantage of the standard ISO procedure.
Learn more about our Robust Design and Reliability technology
Manage uncertainties efficiently and deliver high-quality products
Learn more about our Robust Design and Reliability technology
Manage uncertainties efficiently and deliver high-quality products
Learn more about our Robust Design and Reliability technology